Written by Álvaro González Vila (IKERLAN Technology Research Centre, Basque Research and Technology Alliance - BRTA)
Nowadays, we live in an increasingly connected world, where more and more people have access to the internet through the use of computers, smartphones or tablets, among others. Additionally, devices of different nature are connected to the internet by themselves, without requiring human-to-human or human-to-computer interaction, shaping what we currently know as Internet of Things, or IoT [1]. The use of these devices to carry out some autonomous computation has been possible thanks to the progressive increase in computing capacity, leading to powerful mobile or Edge Computing. Consequently, Fog Computing architectures have emerged during the recent years, distributing the computational load in both the Edge devices and the devices in between the Edge and the Cloud, such as switches, routers, workstations.
Fog Computing brings the benefits of Edge and Cloud computing together [2], with the Edge devices continuously growing in terms of computing capability and the Cloud infrastructures having matured substantially both in availability and scalability. While Fog Computing has been in the spotlight by both the research and industry communities, just its foundations have been named, being the holistic implementation of the concept still missing.
There are many scenarios where Edge Computing is crucial [3], such as assisted-driving systems, medical monitoring, smart structures, etc. Fog Computing reduces the amount of data traditionally interchanged between these devices and the Cloud or datacenter, being able to work with unreliable network connections and reduce the information latency to fulfill the final user requirements, i.e. for real-time applications. As shown in the Figure [4], the Fog Computing architecture could be schematically located in an intermediate layer between the Edge devices and the Cloud infrastructure, consequently bringing the computing capabilities closer to the devices themselves. The aim of ELASTIC is therefore obtaining the best from this kind of architecture and demonstrating its application on a Smart Mobility use-case scenario.
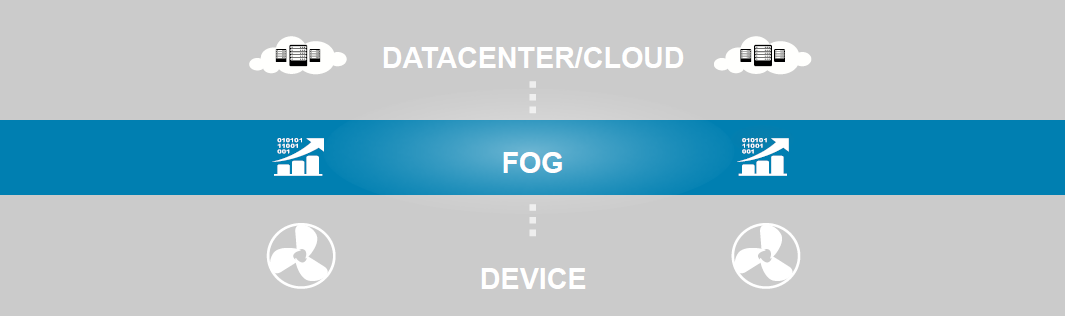
Overview of Fog Computing architecture. Obtained from [4].
In a traditional IoT architecture, data is moved from the devices to the Cloud and, when required, decisions are sent back from the Cloud to the devices, which is generally known as Data Movement. Fog Computing extends this perspective with the concept of Computation Movement, being some processes executed directly in the devices while others are sent to intermediate computing resources to finally leave the most demanding ones to be computed in the Cloud. Dynamic data and processing management enables a distributed schema at different levels of the Fog architecture, allocating resources according to different criteria. This is complemented with storage capability provided in all computing resources, with the possibility of using distributed storage solutions as well [5].
Among its benefits, Fog Computing makes explicit use of various computing layers, each of them with different capabilities. Layers close to the devices exhibit faster response times, providing the closest to real-time computation. In a complementary manner, the Cloud offers higher computing resources, which can be used for heavier tasks. In the meanwhile, computing nodes from the intermediate layers can carry out a variety of tasks. The elasticity concept reduces network usage, improves the response times, and allows the fulfilment of non-functional requirements, providing an added value for the full architecture.
Some of the requirements that will be satisfied in the Fog Computing architecture proposed in the ELASTIC Software Architecture and for the specific scenarios of the Smart Mobility use-cases in the city of Florence will be the following:
- The Fog Computing architecture will have at least three layers: the sensor layer, the station layer, and the Cloud. Information will flow between the layers for distributed storage, processing and decision making.
- The Edge nodes will be able to temporarily store and associate different kinds of data gathered locally by physically connected devices. By doing so, a data buffer will be available in case an issue arises, either at the node or the network side.
- The system will provide long term storage for big data in the cloud, in order to perform demanding data analytics and preserve historical data.
- Part of the obtained data will be transferred to the system to be stored and associated with global data, such as videos or maps, so data consistency will be achieved.
- The system will synchronize data between architectural levels without harming performance, by transferring only the necessary data and only when required.
References:
[1] P. Asghari, A.M.Rahmani, H.H.S. Javadi, “Internet of Things applications: A systematic review”, Computer Networks 148, 241-261 (2019). DOI: 10.1016/j.comnet.2018.12.008
[2] C. Puliafito, E. Mingozzi, F. Longo, A. Puliafito, O. Rana, “Fog computing for the internet of things: A survey”, ACM Transactions on Internet Technology (TOIT) 19(2), 1-41 (2019). DOI: 10.1145/3301443
[3] W.Z. Khan, E. Ahmed, S. Hakak, I. Yaqoob, A. Ahmed, “Edge computing: A survey”, Future Generation Computer Systems 97, 219-235 (2019). DOI: 10.1016/j.future.2019.02.050
[4] Cisco Systems, Inc., USA, “Fog Computing and the Internet of Things: Extend the Cloud to Where the Things Are”, White Paper, April 2015. URL: https://www.cisco.com/c/dam/en_us/solutions/trends/iot/docs/computing-overview.pdf
[5] J. Martí, A. Queralt, D. Gasull, A. Barceló, J.J. Costa, T. Cortes, “Dataclay: A distributed data store for effective inter-player data sharing”, Journal of Systems and Software 131, 129-145 (2017). DOI: 10.1016/j.jss.2017.05.080